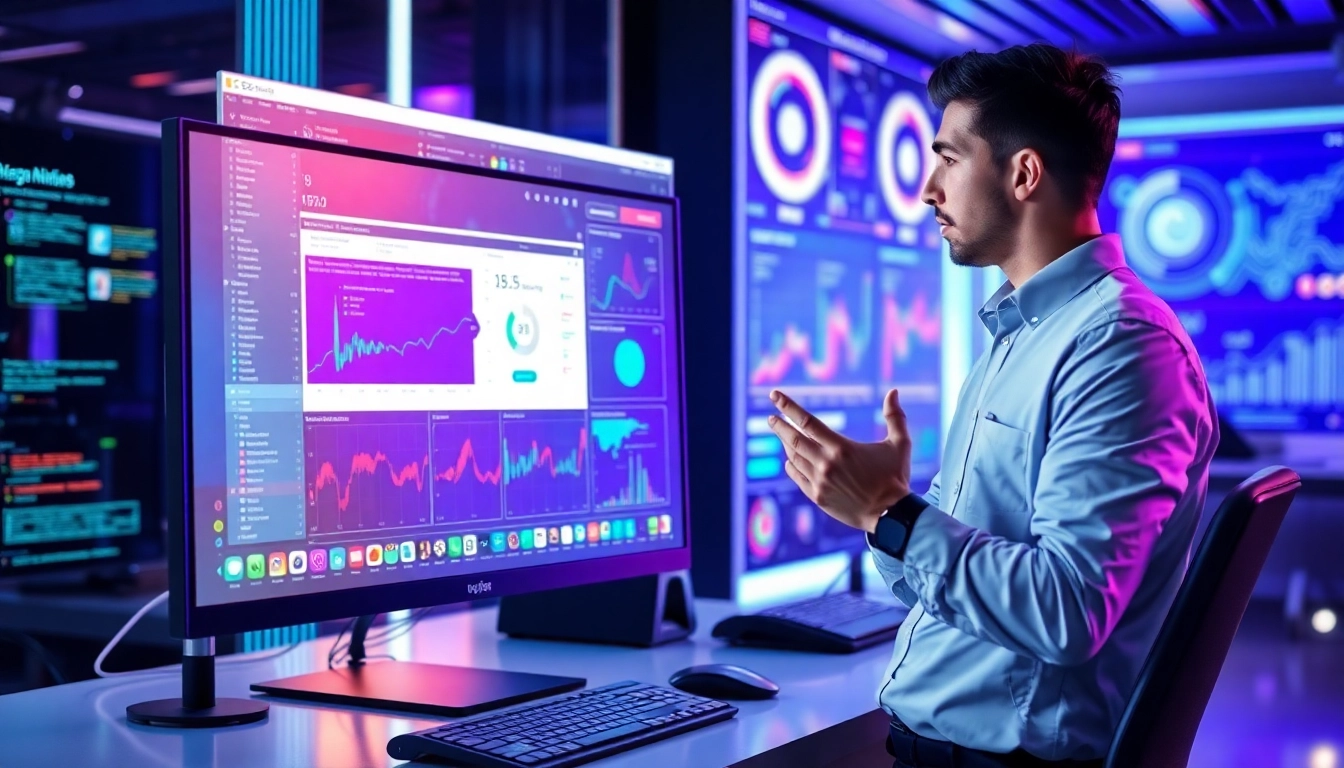
Understanding AI Sentiment Analysis
Defining Sentiment Analysis in the Age of AI
Sentiment analysis, also known as opinion mining, is a branch of natural language processing (NLP) that focuses on evaluating and interpreting human emotions expressed in text. In the modern, digital landscape, sentiment analysis has evolved from traditional methodologies to advanced AI-driven approaches. Through the application of machine learning, sentiment analysis can automatically determine whether the sentiments expressed in a piece of text are positive, negative, or neutral. By utilizing vast datasets from various sources, organizations can gain insights into public perception, customer satisfaction, and overall sentiment trends. As we advance further into the age of artificial intelligence, AI sentiment analysis is proving to be an essential tool for data-driven decision-making.
Importance of Sentiment Analysis for Businesses
For businesses operating in today’s competitive environment, understanding customer sentiment has become crucial. Insights gleaned from sentiment analysis can effectively inform marketing strategies, product development, and customer service initiatives. Companies can leverage this data to identify areas for improvement, respond proactively to negative sentiments, and enhance overall customer satisfaction. As organizations strive to foster a customer-centric approach, sentiment analysis provides them with the capability to tailor their offerings based on customer feedback and emotional responses. Moreover, businesses can utilize sentiment analysis to gauge public opinion about brands, competitors, and emerging trends in their industry.
How AI Transforms Traditional Methods
Traditionally, sentiment analysis involved manual processes or rudimentary algorithms, which often yielded inconsistent and unreliable results. AI technologies, particularly machine learning algorithms, have transformed sentiment analysis by enabling more accurate and efficient processing of large datasets. AI can recognize subtle nuances within the text, contextual meanings, and even the emotional intensity of sentiments expressed. This transformation integrates advanced techniques like deep learning, which allows machines to learn from vast amounts of text data, resulting in refined models that enhance the accuracy of sentiment classifications. Furthermore, AI can automate the sentiment analysis process, allowing organizations to analyze customer sentiment in real-time across various channels, such as social media, online reviews, and customer feedback surveys.
The Process Behind AI Sentiment Analysis
Data Collection Techniques
The success of AI sentiment analysis relies heavily on the quality and comprehensiveness of the data collected. Various techniques can be employed to gather data, including web scraping, usage of APIs, and direct user feedback collection. Organizations often collect data from multiple sources, catering to diverse platforms where customers express their thoughts, such as:
- Social Media Platforms: Gathering insights from platforms like Twitter, Facebook, and Instagram to analyze customer sentiment towards brands or products.
- Online Reviews: Scraping reviews from sites like Yelp, Amazon, and Google to gauge consumers’ opinions.
- Surveys and Feedback Forms: Directly soliciting feedback from customers through surveys to gather qualitative data on sentiments.
After gathering data, it’s essential to preprocess it for optimal analysis. This process may include removing irrelevant content, handling missing data, and converting text into a usable format for algorithms.
Natural Language Processing Explained
Natural Language Processing (NLP) is a pivotal technology in AI sentiment analysis, enabling machines to understand and interpret human language. NLP encompasses several tasks essential for sentiment analysis, including tokenization, part-of-speech tagging, named entity recognition, and dependency parsing. By breaking down sentences into manageable components, NLP allows AI models to understand the context and sentiment embedded within each text. For instance, NLP can distinguish between sarcasm and genuine sentiment by analyzing the relationships and structures within a sentence.
Machine Learning Algorithms in Sentiment Analysis
Machine learning algorithms play a vital role in sentiment analysis by enabling AI to categorize sentiments based on training data. Several algorithms can be deployed, including:
- Supervised Learning: Requires labeled data to train models on how to categorize sentiments accurately. Common algorithms include Support Vector Machines (SVM), Logistic Regression, and Neural Networks.
- Unsupervised Learning: Involves clustering data without prior labeling, enabling the discovery of hidden sentiment patterns through methods like K-means clustering and hierarchical clustering.
- Deep Learning: Utilizes multi-layered neural networks to improve the accuracy of sentiment classification, especially in handling complex language structures and contexts.
By leveraging these machine learning techniques, businesses can develop models that predict sentiment with a high degree of accuracy, thereby creating actionable insights from data analysis.
Implementing AI Sentiment Analysis Tools
Choosing the Right Tool for Your Needs
Selecting the appropriate AI sentiment analysis tool is crucial in yielding effective results. Vendors offer a variety of tools with different features, capabilities, and cost structures. When choosing a tool, organizations should consider essential factors such as:
- Integration Capabilities: The tool should easily integrate with existing systems, whether it’s CRM software, social media management platforms, or marketing automation systems.
- Customization Options: The ability to customize the tool based on specific business needs, including tailored sentiment dictionaries and training models.
- Scalability: The tool should handle varying data volumes as business demands grow and adapt seamlessly to changing business environments.
- Analytics and Reporting: Comprehensive reporting features allow organizations to extract insights effectively and share data with relevant stakeholders.
Integrating AI Tools into Business Operations
Once the right tool is identified, the next step involves integrating it into the organization’s existing operations. This may include the following steps:
- Conducting a Pilot Test: Testing the selected tool on a smaller, controlled dataset to evaluate its performance and accuracy before a full-scale rollout.
- Training Employees: Providing adequate training sessions for team members on how to use the tool effectively and interpret sentiment insights accurately.
- Establishing Feedback Loops: Creating mechanisms for continuous feedback on the tool’s performance, ensuring ongoing refinement and adjustment.
- Monitoring Performance: Regularly assessing the tool’s output against predefined metrics and KPIs to ensure its effectiveness in meeting business objectives.
Real-Time Data Processing Capabilities
The ability to process data in real-time is a significant advantage of AI sentiment analysis tools. Real-time analysis allows organizations to track customer sentiment trends as they emerge, enabling proactive engagement strategies. This capability is especially beneficial for managing brand reputation during crises or product launches where public sentiment can fluctuate rapidly. By integrating real-time sentiment monitoring, businesses can respond swiftly to customer feedback, addressing concerns and reinforcing positive sentiments, leading to improved customer relations and enhanced brand loyalty.
Common Applications of AI Sentiment Analysis
Sentiment Analysis in Customer Feedback
Customer feedback is a goldmine of sentiment data. By analyzing feedback from surveys, reviews, and social media comments, organizations can gain valuable insights into customer experiences and preferences. Sentiment analysis tools can highlight common themes in customer feedback, identifying areas of satisfaction or concern. For instance, if a significant number of customers express dissatisfaction with a product feature, businesses can take action to modify and enhance the product to better meet consumer expectations.
Using Sentiment Analysis for Market Trends
Beyond direct customer feedback, sentiment analysis can be applied to broader market trends. By assessing how consumers feel about specific products, industries, or brands across various platforms, companies can identify emerging trends that could impact their strategies. This information is invaluable for market research, enabling businesses to tap into new opportunities or pivot their marketing strategies based on shifting consumer sentiment. Companies can even apply sentiment analysis to competitive intelligence, gauging public sentiment about competitors and positioning themselves accordingly.
Enhancing Customer Support with AI
AI sentiment analysis can significantly enhance customer support operations. By integrating sentiment analysis into customer support channels, organizations can assess the emotional tone of customer inquiries and complaints. This information enables support teams to prioritize tickets based on urgency, ensuring timely resolutions for pressing issues. Additionally, understanding customer sentiment can guide support agents in tailoring their responses to better align with the emotional state of the customers they are assisting, leading to more effective interactions and improved customer satisfaction.
Evaluating the Impact of AI Sentiment Analysis
Key Performance Indicators for Success
To measure the effectiveness of AI sentiment analysis implementations, organizations should establish clear Key Performance Indicators (KPIs). Potential KPIs may include:
- Sentiment Accuracy: The percentage of correctly categorized sentiments compared to human evaluations.
- Response Time: The time taken to respond to customer feedback and implement changes based on sentiment analysis insights.
- Customer Satisfaction Score (CSAT): Post-interaction satisfaction ratings reflecting how well support and products meet customer needs.
- Engagement Levels: Metrics on social media and website engagement to assess changes in consumer behavior following sentiment-driven initiatives.
Case Studies: Successful Implementation
Several organizations have successfully implemented AI sentiment analysis to drive results. For example, a retail brand that introduced sentiment analysis tools saw a marked improvement in customer satisfaction. By analyzing customer feedback across social media, they identified common issues related to product delivery. Using these insights, the brand was able to streamline their logistics process, resulting in a 25% improvement in delivery times and increased positive customer feedback. This case illustrates how effective sentiment analysis can directly correlate with enhanced operational efficiency and customer loyalty.
Future Trends in AI Sentiment Analysis
As technology continues to advance, several trends are expected to shape the future of AI sentiment analysis. These include:
- Enhanced Contextual Understanding: Improvements in NLP will lead to better sentiment analysis by enabling machines to grasp complex sentences and emotional subtleties.
- Integration with Voice and Visual Data: Expanding sentiment analysis beyond text to include voice-based analytics and visual content, enabling a more holistic assessment of consumer sentiment.
- Integration of AI and Business Intelligence Tools: Merging sentiment analysis with business intelligence platforms to provide organizations with rich, data-driven insights that inform broader strategies.
Overall, the future of AI sentiment analysis seems promising, with innovations and advancements likely to unlock even more potential for organizations to understand and respond to customer sentiments in real-time.